Machine Learning Latest Updates: Innovations, Trends, and Future Prospects
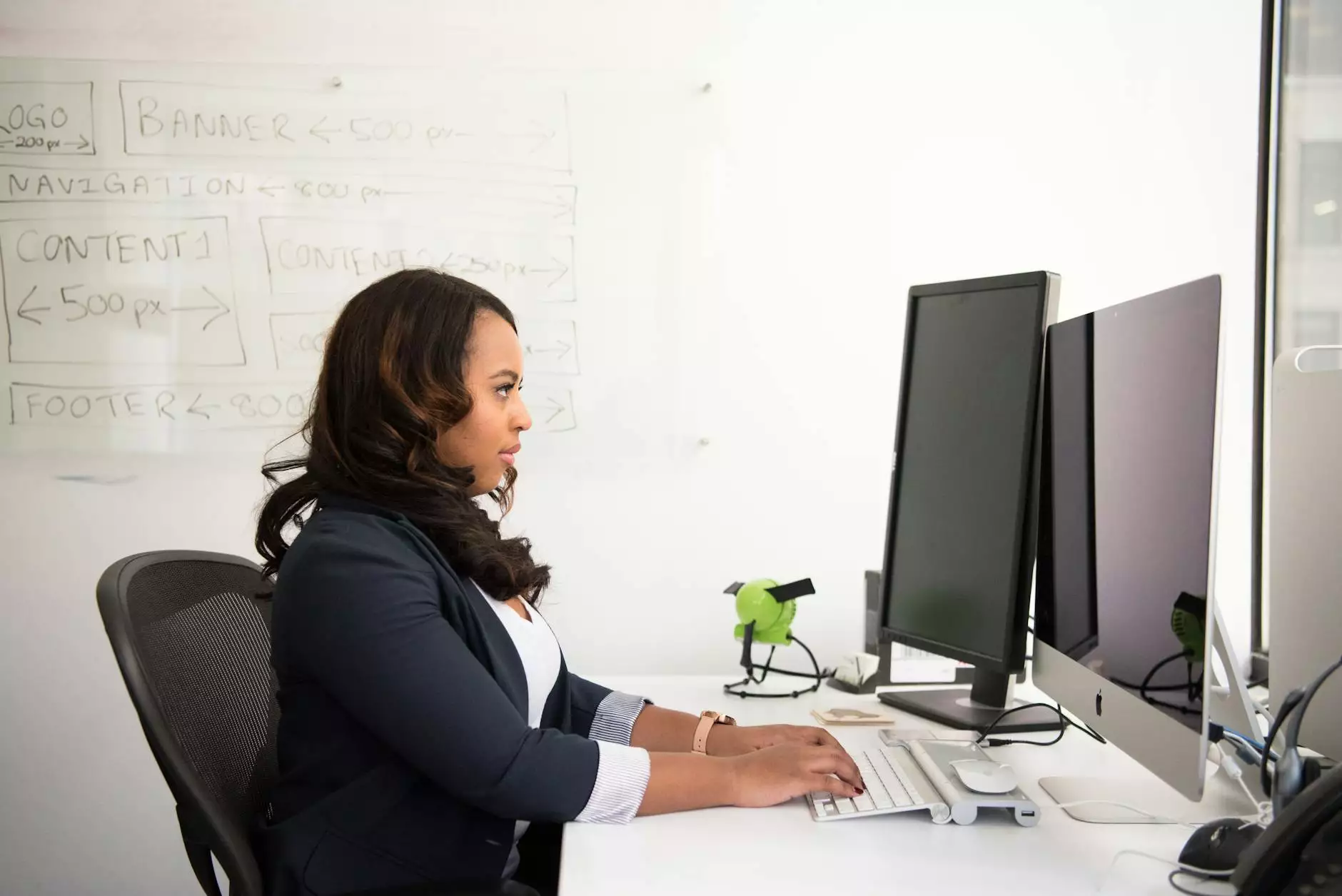
Machine learning has become an indispensable element in modern technology, powering advancements across various sectors from finance to healthcare. As we delve into the machine learning latest updates, we uncover the most significant breakthroughs and trends that are shaping the future of this remarkable field. Our goal is to provide a comprehensive overview of these innovations, showcasing their impact on both businesses and everyday life.
Understanding Machine Learning
Before diving into the latest updates, it's essential to grasp the fundamentals of machine learning. At its core, machine learning is a subset of artificial intelligence (AI) that enables systems to learn from data and improve their performance without being explicitly programmed. Here are some key components that constitute the foundation of machine learning:
- Algorithms: The mathematical models that enable machines to process and analyze data.
- Data: Quality data is vital, as it serves as the training ground for machine learning models.
- Training: The process of feeding data into an algorithm so it can learn to make predictions or decisions.
- Inference: The application of a trained model to new data for making predictions.
Recent Innovations in Machine Learning
The field of machine learning is continuously evolving, with new technologies and methodologies emerging regularly. Here are some of the most notable recent innovations that illustrate the direction in which machine learning is headed:
1. Transformers and Natural Language Processing
The introduction of the transformer architecture has revolutionized the field of Natural Language Processing (NLP). This innovation allows models to better understand context and semantics in human language. As a result, applications such as chatbots, translation services, and content generation tools have seen significant advancements. The latest models, such as GPT-4, provide more coherent and contextually aware responses, thereby enhancing user interaction and experience.
2. Federated Learning
As data privacy becomes increasingly paramount, federated learning emerges as a vital innovation. This decentralized approach allows multiple devices to collaboratively learn a shared model while keeping all data on-device. It enhances privacy while significantly improving learning efficiency. For instance, companies like Google are implementing this technology to train models for their mobile devices without compromising users' personal data.
3. Automated Machine Learning (AutoML)
The complexity of building and deploying machine learning models is often a barrier for many businesses. Enter Automated Machine Learning (AutoML), which simplifies the process by automating the model selection, hyperparameter tuning, and feature engineering tasks. This allows non-experts to harness machine learning capabilities without needing extensive technical knowledge. Platforms like Google Cloud AutoML are leading the charge in making these capabilities accessible to a broader audience.
4. Explainable AI (XAI)
As machine learning models grow in complexity, the need for transparency becomes critical. Explainable AI (XAI) is an emerging field that aims to make the decision-making processes of machine learning models more understandable to humans. This innovation is particularly crucial in industries such as healthcare and finance, where the implications of AI decisions can have significant consequences. By explaining how a model arrived at a particular conclusion, organizations can increase trust and accountability.
Trends Shaping the Future of Machine Learning
The landscape of machine learning is shaped not only by innovations but also by several key trends. Here are the trends that are currently defining the realm of machine learning:
1. Integration of Machine Learning with IoT
The integration of machine learning with Internet of Things (IoT) devices is set to enhance data processing capabilities and decision-making processes. As IoT devices proliferate, the volume of data generated will require efficient analysis. Machine learning algorithms can help in interpreting this data in real-time, providing actionable insights across various domains, including smart cities and energy management systems.
2. Greater Focus on Data Ethics and Governance
With the increasing utilization of data comes the responsibility to manage it ethically. Companies are now placing greater emphasis on developing data governance frameworks to ensure compliance with regulations like GDPR. Ethical considerations such as bias in machine learning models and data privacy are at the forefront of discussions among industry leaders.
3. Enhanced Collaboration Across Industries
As machine learning becomes integral to various sectors, cross-industry collaborations are becoming common. Organizations are pooling their resources and expertise to solve complex problems, such as predictive healthcare and climate modeling. This collaborative effort accelerates the pace of innovation and expands the application of machine learning technologies.
4. Continuous Learning and Adaptability
In a fast-changing technological environment, there is a growing trend towards developing models that can learn and adapt continuously. This capability, often referred to as online learning, allows machine learning systems to update themselves in real-time based on new data, enhancing their robustness and relevance. Companies are particularly interested in this approach for applications such as fraud detection and predictive maintenance.
Challenges Facing Machine Learning Adoption
Despite its numerous advantages, the adoption of machine learning is not without challenges. Recognizing these obstacles is essential for businesses looking to implement machine learning solutions effectively. Here are some of the primary challenges:
1. Data Quality and Availability
The effectiveness of machine learning models is heavily reliant on data quality. Inaccurate, incomplete, or biased data can lead to misleading outcomes. Additionally, the unavailability of sufficient data can hinder the training process, especially for complex models. It is imperative for organizations to invest in data management strategies that ensure high-quality data is accessible.
2. Talent Shortage
There is a significant shortage of skilled professionals who can design, implement, and maintain machine learning systems. As demand for machine learning expertise grows, companies are competing to attract top talent. Organizations may need to consider alternative approaches, such as partnership with educational institutions and offering training programs for existing employees.
3. Integration with Legacy Systems
Many organizations struggle with integrating modern machine learning applications with their existing legacy systems. This can create silos of information and limit data accessibility. A strategic approach to digital transformation, which involves updating legacy systems and ensuring interoperability, is crucial for leveraging machine learning effectively.
4. Regulatory Compliance
As machine learning technologies evolve, so do the regulatory environments surrounding their use. Organizations must navigate complex legal frameworks that govern data usage and privacy. Ensuring compliance while still harnessing the full potential of machine learning is a critical challenge that requires ongoing attention and resources.
The Future of Machine Learning: Opportunities Ahead
Looking forward, the future of machine learning is filled with opportunities that can transform various sectors. Here are potential avenues for growth and innovation:
1. Personalized Customer Experiences
Machine learning has the potential to revolutionize the way businesses understand and interact with their customers. By leveraging data analysis, organizations can create highly personalized experiences that resonate with individual preferences. This approach not only enhances customer satisfaction but also drives loyalty and revenue growth.
2. Smart Automation in Manufacturing
The manufacturing sector stands to benefit significantly from machine learning advancements, particularly in the area of automation. Predictive maintenance powered by machine learning algorithms can reduce downtime and optimize production processes. Additionally, smart factories that utilize machine learning can improve efficiency and safety.
3. Advances in Healthcare Diagnostics
Machine learning is poised to make remarkable strides in healthcare, particularly in diagnostics. With the ability to analyze vast amounts of medical data, machine learning algorithms can assist in early disease detection and personalized treatment plans. This could lead to improved patient outcomes and reduce healthcare costs in the long run.
4. Environmental Sustainability
As global awareness of environmental issues rises, machine learning can play a crucial role in sustainability efforts. From optimizing energy consumption to enhancing resource management and reducing waste, the applications of machine learning in environmental conservation are vast and impactful.
Conclusion
The machine learning latest updates reveal a field that is not only dynamic but also essential for future technological advancements. Organizations across various sectors must stay informed about these developments to leverage machine learning effectively for competitive advantage. By embracing innovations, understanding emerging trends, and addressing challenges head-on, businesses can harness the full potential of this transformative technology. As we look towards the future, the continued evolution of machine learning promises exciting possibilities for innovation and growth.